思科数据科学家如何帮助普通的创新教师反馈以获得更好的学习
By Kyle Thornton
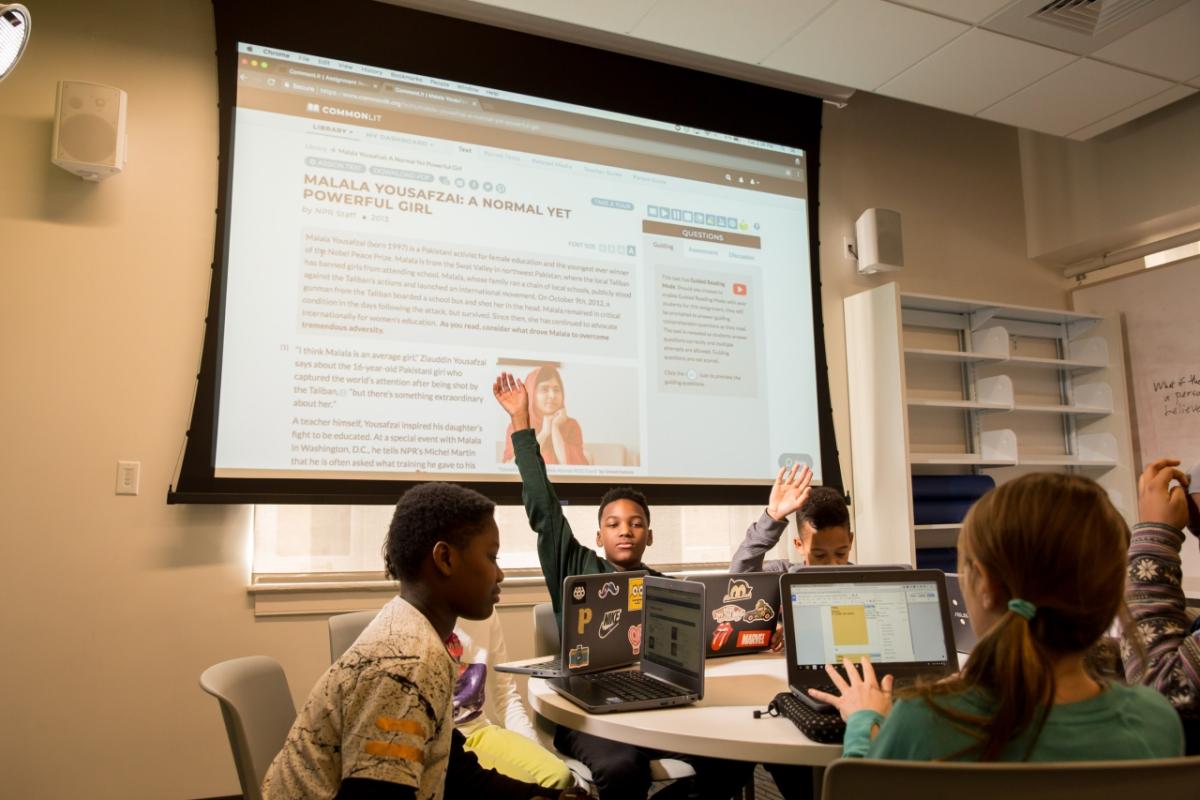
学生阅读和数学理解正在下降。美国。 ”国家记分卡,”基于长期分数,由全国教育协会(NAEP)记录,并由国家经济研究局(NEBR),显示自1990年以来阅读的平均得分下降最大,并且是数学有史以来的第一个得分下降。这种下降来自对49个州10,000所学校中200万名学生的测试数据的分析。
More can and will be done to address the implications of widening achievement for all students, and in particular students from underserved districts. To stimulate an academic recovery, we need innovative classroom solutions like CommonLit to support teachers and their students in today’s classrooms.
For years, Cisco nonprofit granteeCommonLit专注于他们的使命,以帮助学生学习如何成为更好的读者和作家。他们在方法方面取得了成功:通过让学生在线获取阅读材料,作业和测试的访问,并通过为教师提供资源,例如仪表板,这些仪表板显示了孩子们可能在某些技能上挣扎的地方。
“CommonLit offers programs that are fully interactive and have everything teachers and students need—much like a reading program in a box.” Agnes Malatinszky, Chief Operating Officer at CommonLit explains.
他们于2019年7月推出的高度吸引人的注释工具使教师能够向学生提供相关和实时的反馈。但是研究表明,及时接收反馈会带来更好的学生成果。因此,CommonLit的团队希望找到方法,使他们的注释工具更有效地供老师使用。
So two years ago, with support from the思科基金会, CommonLit came to Cisco’s data scientists, who volunteer with AI for Good, to help them review Annotation Tool usage information and determine ways to optimize the Annotation Tool through machine learning (ML) to help teachers and students better connect.
Partnering up and giving back
在思科,我们拥有通过战略社会影响赠款以及强烈的回馈文化来支持非营利组织的良好记录。思科的AI for Goodprogram brings these values together by connecting Cisco data science talent to nonprofits, like CommonLit, that do not have the resources to use AI/ML to meet their goals.
This CommonLit and AI for Good partnered project was led by data scientist Kirtee Yadav, who also served as cause champion–which means she led the project from start to finish to ensure the project’s success. Other members of the project included Technical Lead Sampann Nigam and Team members, William Bickelmann, Bob Lapcevic, Aakriti Saxena, Sree Yadavalli, and Tana Franko.
Cisco的客户体验产品经理Kirtee Yadav说:“这个共同的项目是Cisco的数据科学家通过使用独特技能来帮助有效理由的好机会。”“我抓住了这个机会,因为它为我提供了对这个非营利组织产生影响的新技能的机会。”
通过数据科学找到差距
Multiple studies已经表明,孩子收到的反馈越多,完成作业后收到的速度就越快,他们互动和从内容中学习的越多。然而,许多老师通常没有时间提供详细的个性化反馈。
CommonLit challenged AI for Good data scientists to find out how that issue can be improved or resolved. The first thing the AI for Good team did was look at how Cisco’s machine learning models could modify, streamline, or improve the Annotation Tool so teachers could more efficiently give feedback to students. Through results from pulled data, they found that teachers could only provide feedback to an average of two percent of student’s comments.
“Based on our analysis of the [CommonLit] data,” Sampann Nigam, data science leader at Cisco and tech lead on the AI for Good team, pointed out, “we found that feedback from teachers pushes engagement up. So, we created an AI model to recommend feedback options to teachers.”
The data science-built solution
经过几个月的研究和努力,优秀团队的AI建立了自然语言处理(NLP)解决方案,以帮助带宽有限的教师向更多的学生提供反馈。通过NLP,改进的注释应用程序将生成三个建议的反馈短语,并增加了自由形式反馈的选择。
“The feedback is built to look like teacher’s direct feedback, but instead it’s a tool that provides feedback options, which teachers can pick and send to students with just a click,” Kirtee reasoned. “In the end, AI for Good helped CommonLit improve their student teacher feedback loop.”
Sampann对我们描述了技术过程:良好团队的AI使用BERT(来自变形金刚的BI向编码器表示)模型和自由形式反馈预测,使用T5模型构建了短语预测解决方案。数据科学家通过使用带注释的文本和学生注释的数据集作为特征集和反馈短语作为标签培训(微调)BERT模型。
“Besides generating a set of phrases as suggested feedback, we decided to provide freeform feedback,” Sampann said. “These models use transfer learning, a specialized machine learning process.”
成功证明
超过一百万的教师在80,000多所学校中使用普通单位。到2019年秋季学期,就在推出仅几个月后,超过603,000名学生使用了注释工具,创建了3,210,156个注释和5,029,973个亮点。因此,毫不奇怪的是,通过新近改进的自然语言处理的注释工具在第一年就非常有用 - 记录了接近250万个注释。
“像CommonLit这样的教育技术工具永远无法取代课堂老师,但我们可以使他们的时间更加有效。”艾格尼丝说:“我们对机器学习和自然语言处理工具的思考方式,例如为注释工具开发的,是为了使教师的工作尽可能容易。我们可以使他们的工作更轻松。我们可以将他们推向最佳实践。”
This data science focused collaboration included a team of ten AI for Good data science volunteers and over 200 hours of their time spent on research, analysis, and problem-solving to successfully build a natural language-informed Annotation Tool.
The AI for Good team is working with CommonLit on options for releasing their Feedback recommendation AI model as open source, so that the education community can benefit from it.
“Cisco has been a partner for us for a couple of years now. They have supported some of our most innovative work around the technology,” Agnes explained. “And working with the Cisco AI for Good team was a unique experience that moved our organization’s most cutting-edge work forward.”
Learn more about our partnership withCommonLit.
View original content这里。